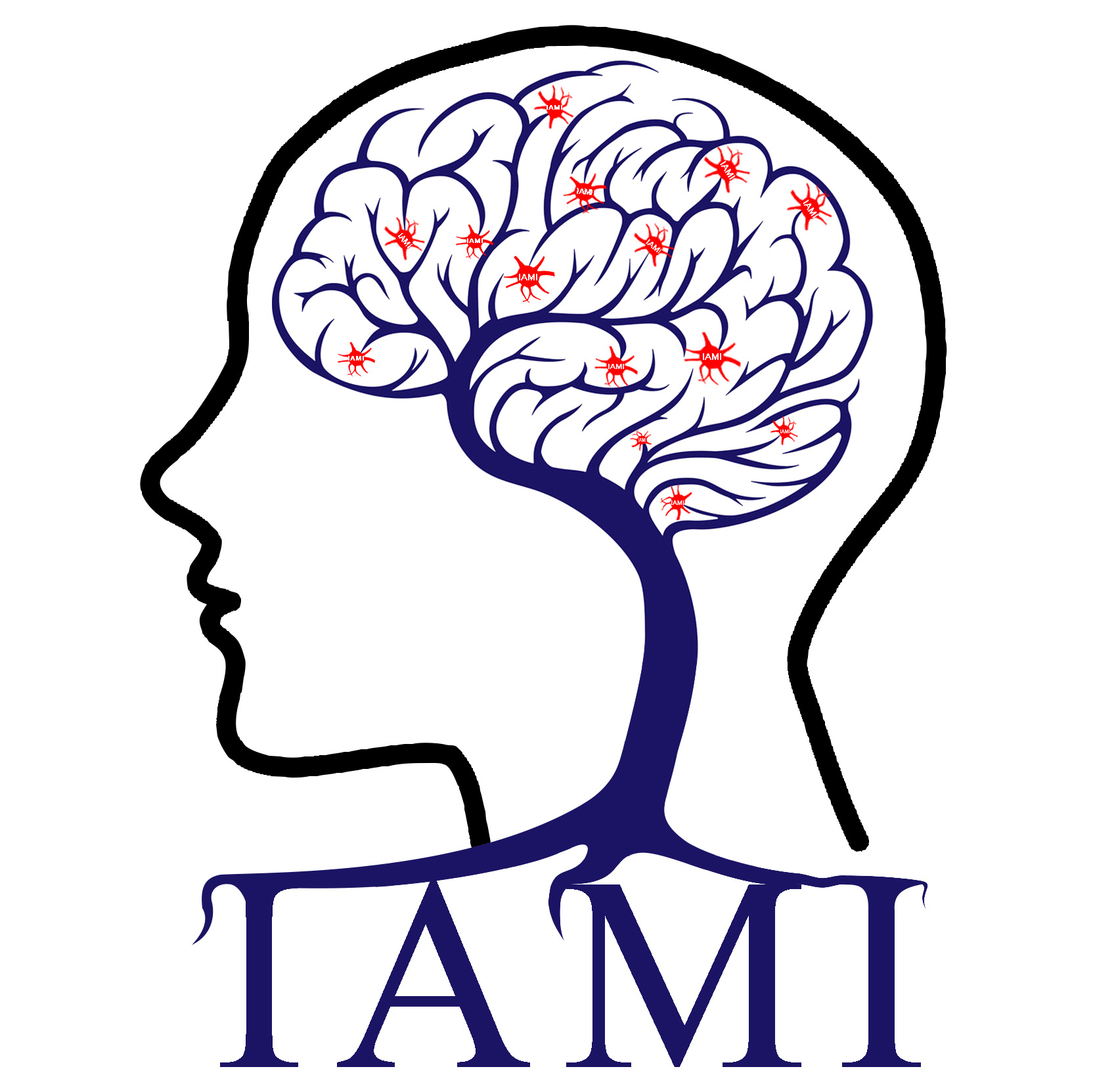
Yuhui Du Personal Website-Intelligent Analysis of Medical Image
Address:Taiyuan, China
Yuhui Du*, Yuliang Hou, Yu Zhang, A New Spatio-Temporal Joint Attention-Based Deep Learning Model for Decoding Brain Cognition Function, China Automation Congress (CAC), 2023, 6965-6970
时间:2024-04-15 17:06:41 来源: 点击:[363]
Abstract:
Decoding different brain cognitive states using neuroimaging techniques such as functional magnetic resonance imaging (fMRI) can greatly enhance our understanding of how the brain works and facilitate the development of brain-computer interfaces and brain-like intelligence. Although many AI methods are used to identify patterns of neural activity that are associated with specific cognitive states, how brain regions interact over space and time in different cognitive states is still largely unknown. In this study, we propose a new deep learning model that incorporates a spatio-temporal joint attention for decoding brain cognitive states from fMRI data. In particular, long short-term memory (LSTM) modules are employed in our model to efficiently handle variable-length sequential data, which enables our method to work for the condition in which different states involve different lengths of data. Using the large-sample (n=41,796) datasets from Human Connectome Project, our proposed model achieves better performance (92.59% accuracy) in classifying 23 complex cognitive states, compared to the traditional machine learning and deep learning methods including support vector machine (SVM), multilayer perceptron (MLP), gate recurrent unit (GRU) and graph convolutional network (GCN). Furthermore, the spatio-temporal features extracted through the spatio-temporal attention are greatly associated with brain cognitive states. In summary, our proposed method is capable of revealing the integrated spatial and temporal information of neural activities, advancing brain decoding research toward a more comprehensive understanding of cognition.