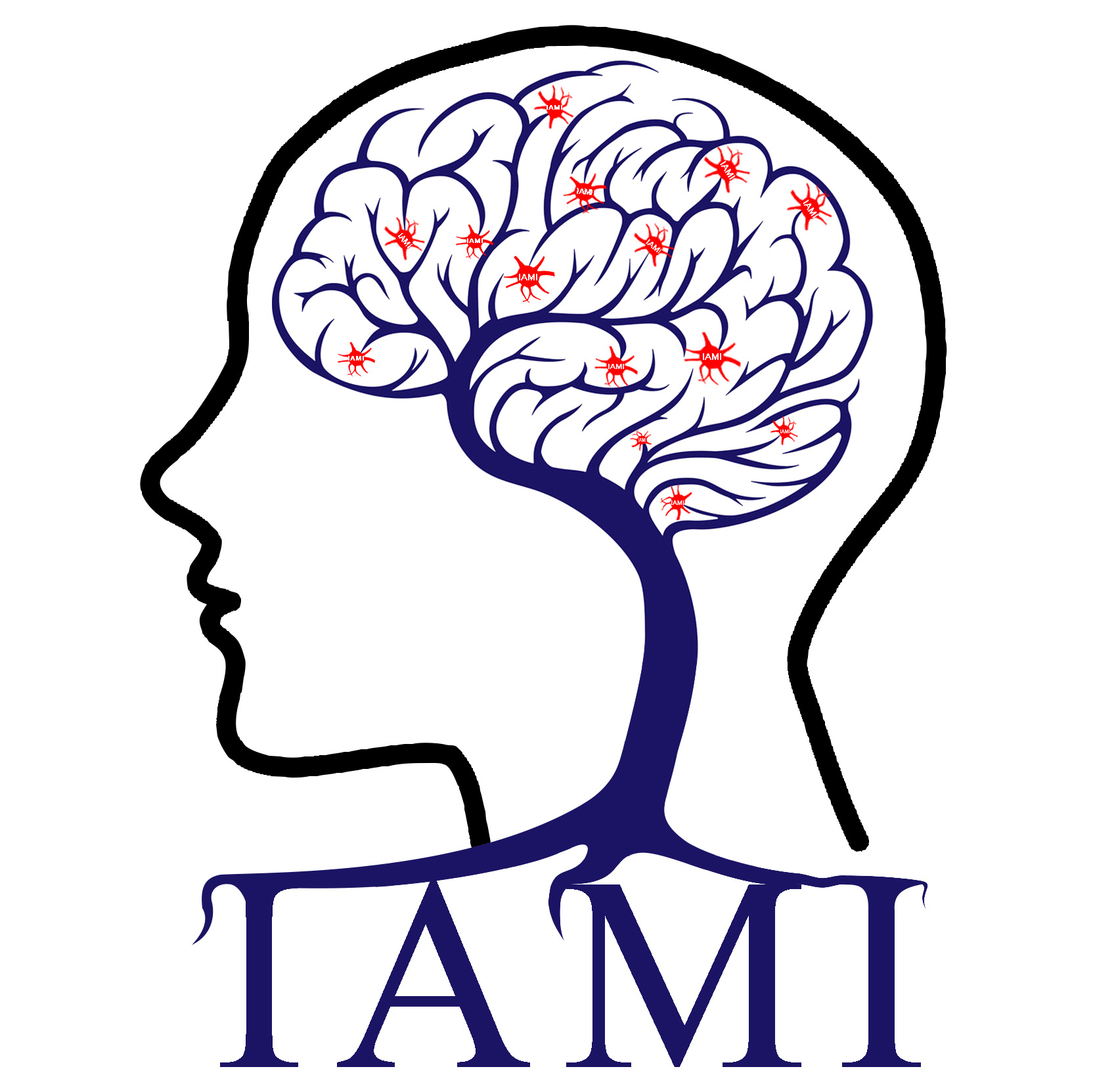
Yuhui Du Personal Website-Intelligent Analysis of Medical Image
Address:Taiyuan, China
Zening Fu, Yuhui Du, Vince Daniel Calhoun. The Dynamic Functional Network Connectivity Analysis Framework. Engineering, 2019, 5(2): 190-193.
时间:2020-06-19 20:59:40 来源: 点击:[804]
Overview
The human brain is a highly dynamic system with time-varying neural activity and rapidly changing neural integration [1–3]. Research using high-temporal-resolution imaging techniques (such as electroencephalography, EEG) has identified reoccurring microstates (by characterizing changes in the power of brain activity) [4,5], which are related to spontaneous thoughts and mental processes [6]. Functional magnetic resonance imaging (fMRI) is a technique that measures brain activity by detecting brain changes associated with blood flow. fMRI has relatively low temporal resolution (i.e., the sampling rate is usually around 0.5), and most previous fMRI analyses employed the static functional connectivity (sFC) between regions of interest, or its independent component analysis (ICA) analog [7], the static functional network connectivity (sFNC) between intrinsic connectivity networks [8–10], in which the potential transient dynamics in brain integrations were not evaluated. Emerging evidence suggests that characterizing the dynamic functional connectivity (dFC) and the dynamic functional network connectivity (dFNC) may advance our understanding of how large-scale functional brain organization changes to support different fundamental functions and behavior, although there are still limitations on the analysis techniques and interpretations [11–17].
To date, sliding window approaches are the most commonly used strategy to characterize dFC from resting-state data [2], although many other methods have been applied [18–21]. Based on the sliding windowed functional connectivity (FC) patterns, it is possible to use clustering and decomposition methods to identify the highly replicable, transient connectivity patterns. The Mind Research Network (MRN) developed a dFNC analysis framework that incorporates different methods including clustering and decomposition (spatial and temporal ICA) to extract connectivity states from sliding-window-based dynamic patterns [1,12,22]. This framework comprises two analysis strategies: hard clustering state (HCS) analysis and fuzzy meta-state (FMS) analysis [1,23–25]. The HCS analysis is capable of assessing reoccurred discrete functional network connectivity (FNC) states. The basic idea of HCS analysis is the assumption that a resting-state functional brain network would vary among different brain states with distinct FNC patterns during the scan time. The FMS analysis is used to compute highlevel state-space metrics with the hypothesis that a resting-state functional brain network may be formed by overlapping different FNC patterns. The details of the dFNC analysis framework are provided in Fig. 1. During the past few years, an increasing number of studies have applied these two methods to different fMRI datasets and have identified significant and interesting dynamic patterns in brain connectivity, which provide greater insight into fundamental properties of the functional brain network.
Critical issues
Window size
A key issue of the dFNC analysis framework is how to select an appropriate window size for the optimal estimation of real fluctuations in the resting-state dFNC. Generally, the window should not be too large to over smooth out valuable dFNC variations and not too small to introduce spurious dynamics from the estimation. Studies have suggested that window size ranging from 30 to 60 s can provide a robust estimation of the dynamic fluctuations in resting-state dFNC [1,2,26]. However, some studies also challenging that such a short window size cannot capture real FNC fluctuations [27]. Also, a fixed window size was usually used for the estimation of dFNC across the whole scan. Considering that the rest brain might enter into different mental states with different length of time (for example, functional states identified by the dFNC analysis framework can have significantly different mean dwell time and occurrence rate), a variable window size would be better for capturing the transient dynamics. In statistics, the estimation of the optimal window size at each time point is not trivial because it stems from the fundamental bias-variance tradeoff problem. Fu et al. [28] have introduced a local polynomial regression (LPR) method to estimate the dFC between brain regions. This method is capable of choosing different optimal window size for each time by minimizing the mean square error locally. They applied this novel method into a task-related fMRI dataset and successfully detected interesting transient dynamic patterns in FC. Another alternative to the fixed window size is the dynamic connectivity regression (DCR) method [29]. Unlike the sliding window approach, this method estimates the temporal change point and estimate the functional relationships between brain regions, for data in the temporal partition that falls between pairs of change points [29].
Physiological noise and non-stationary brain activity
Interpretation of the observed dFNC patterns is also a challenging problem. FNC dynamics can be due to the low signal-to-noise ratio (SNR) at each time window [2]. Previous fMRI datasets generally have repetition time (TR) around 2 s. Assuming that one applies the dFNC analysis framework with window size around 30 to 60 s on an fMRI dataset with TR = 2 s, the dFNC are estimated based on relatively few time points (around 15–30 time points) and thus the results are very sensitive to noise. Nowadays, with the development of new technologies, increasing datasets are scanned with shorter TRs and have higher SNR levels. The human connectome project (HCP) is a consortium of sites who aims to map ‘‘human brain circuitry in healthy adults using cutting-edge methods of noninvasive neuroimaging”.y It provides an open source dataset with a high temporal resolution (TR = 0.72 s) which includes more 1000 healthy subjects. Variations in the magnitudes and variance of the blood-oxygen-level-dependent (BOLD) signals also influence the estimation of resting-state dFNC [30,31]. Previous studies have shown that the BOLD signals may be non-stationary with time-varying properties [32,33]. Such time-varying properties can be a consequence of changing levels of SNR, which might further result in the fluctuations of dFNC estimated using a sliding window approach. A recent study introduced an analysis framework based on the sliding window approach for investigating the relationship between the dynamics in brain activity and the dynamics in dFNC [30]. They found that the amplitudes of low-frequency fluctuations (ALFF) of brain networks co-vary with the dFNC across time during the resting-state. Other sources of noise in fMRI, such as scanner drift, cardiac pulsation, and head motion, might also cause nonneural fluctuations in dFNC. Great effort has been made to reduce these non-neural contributions to the brain signals so as to maximize estimated neural-origin dynamics. For example, in our previous studies using the dFNC analysis framework, three head motion correction steps were included. First, during the pre-processing, the fMRI data are head motion corrected using the statistical parametric mapping (SPM) toolbox and six head motion parameters are estimated. Second, after identifying resting-state networks (RSNs) and their corresponding time courses using group ICA, the six head motion parameters are regressed out from the time courses. Third, the mean frame-wise displacement (FD) is estimated based on the head motion parameters and is used as a covariate in the statistical analysis [34].
It is necessary to investigate dFNC and measurements of neural or physiological processes simultaneously so as to understand the neural-origin of these dynamic patterns. Studies have continued to focus on this, and have provided mounting evidence showing potential correlates of dFNC in simultaneously recorded electrophysiological data [11,35,36]. A recent study used a dataset with simultaneously measured EEG and fMRI data to explore whether FNC states correspond to electrophysiological signatures [11]. The study not only identified functional states similar to that observed in previous studies in an independent sample but also showed that FNC changes are highly associated with EEG spectral signatures.
Reliability and replicability
Another question is whether dFNC variation can be modeled best by a multi-stable state space, which assumes that multi-stable connectivity patterns influence the dFNC in time. Since the dFNC analysis framework is a data-driven approach, some dynamic patterns (such as the number of states and the FNC patterns of each state) are not so consistent across different datasets. For example, in Ref. [1], seven reoccurring functional states were identified in healthy subjects, but in Refs. [34,37], only five functional states were observed from a resting-state dataset including both healthy controls and schizophrenia patients. Several reviews have mentioned the need to examine the reliability of dynamic patterns captured by the dFNC analysis framework [12,38]. Studies have begun to investigate the replicability of dFNC patterns in large resting-state fMRI samples [1,23]. By evaluating the HCS method and the FMS method on an fMRI dataset with extremely large sample size (n = 7500), a recent study demonstrated that the dFNC analysis framework captures highly replicated and reliable dynamic patterns in resting-state FNC [23]. Abrol et al. [23] also conducted a surrogate dataset analysis showing that the observed dFNC patterns are indeed statistically significant. The overall results demonstrated that the dFNC analysis framework is robust against variation in data quality, analysis, grouping, and decomposition methods.
Alternative approaches
There are also many variations on the framework for the exploration of dynamic patterns in the resting-state, such as hidden Markov models that estimate probabilities between gradual transitions [18], change point models that focus on transition points [21], dynamic coherence analysis that offers a data-driven window using wavelets [19], windowless analysis [20], co-activation pattern analysis [31], flexible least-squares-based time-varying parameter regression strategy [39], and independent vector analysis to capture spatial variations over time [16]. Each of these approaches appears to offer a complementary perspective on time-varying connectivity in resting-state fMRI.
Conclusion
The dFNC analysis framework is a novel and powerful technique for the exploration of dynamic patterns in resting-state FC. Comprehensive studies in both real and surrogate datasets demonstrate this framework to be a robust tool for characterizing reliable and highly replicated dFC features. This framework has been applied in many psychiatric disorder studies and identifies numerous atypical patterns in dFC that may serve as candidates for neuroimaging biomarkers within heterogeneous clinical populations. Taken together, these findings support and expand previous knowledge regarding static brain connectivity and atypical brain connectivity in disorders, and strongly suggest the use of the dFNC analysis framework for better improving our understanding of brain functional mechanisms.
Acknowledgements
This work was supported by the National Institutes of Health grants (R01EB006841, R01REB020407, and P20GM103472 PI: VC),the National Natural Science Foundation of China (61703253), and the Natural Science Foundation of Shanxi Province (2016021077).