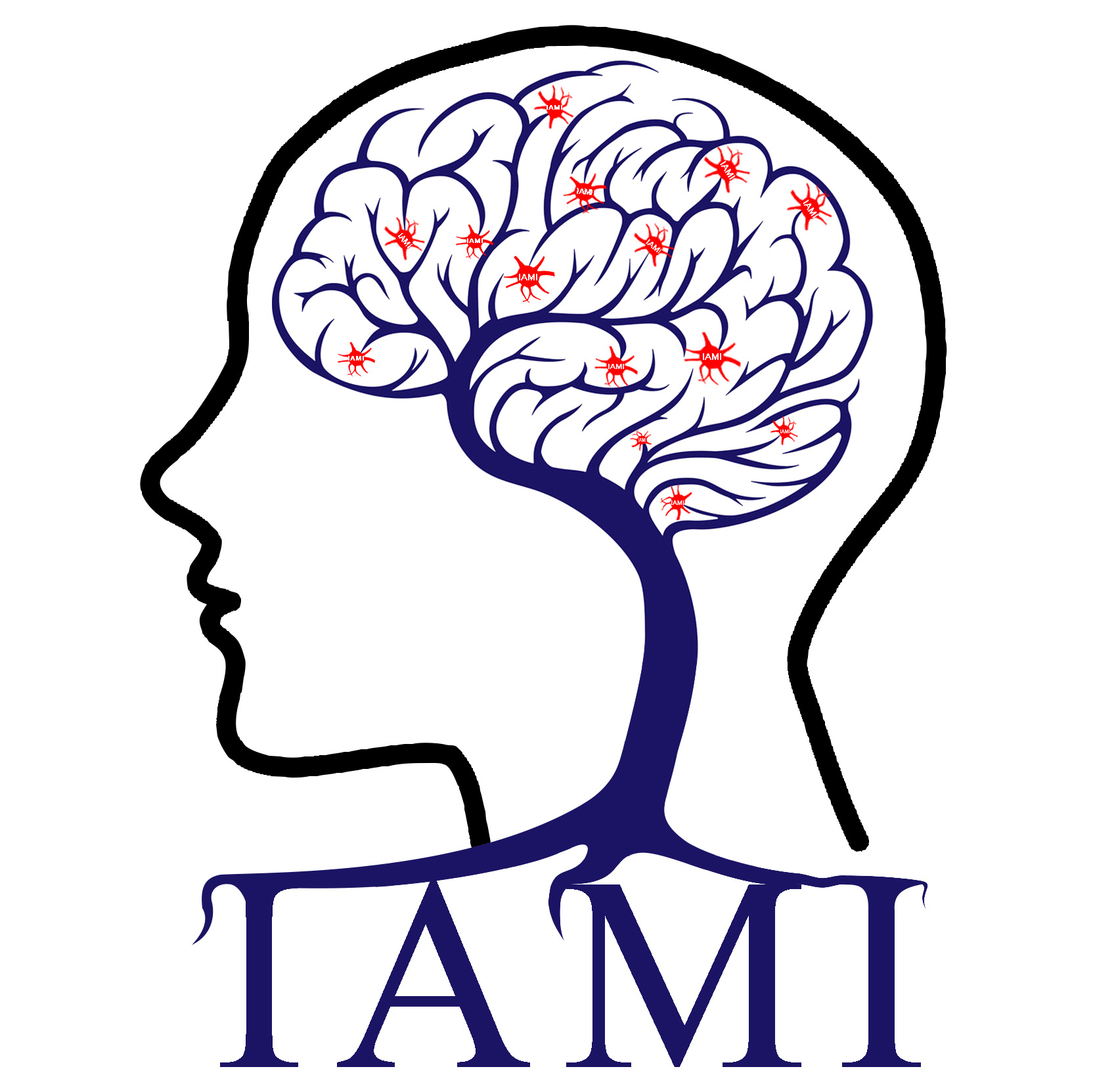
Yuhui Du Personal Website-Intelligent Analysis of Medical Image
Address:Taiyuan, China
Armin Iraji, Ashkan Faghiri, Noah Lewis, Zening Fu, Thomas DeRamus, Shile Qi, Srinivas Rachakonda, Yuhui Du, Vince Calhoun. Ultra-high-order ICA: an exploration of highly resolved data-driven representation of intrinsic connectivity networks (sparse ICNs). Wavelets and Sparsity XVIII. International Society for Optics and Photonics, 2019, 11138: 111380I.
时间:2020-06-19 19:51:58 来源: 点击:[1032]
Abstract
Spatial independent component analysis (sICA) has become an integral part of functional MRI (fMRI) studies, particularly with resting-state fMRI. Early work used low-order ICA with between 20 and 45 components, which has led to the identification of around a dozen reproducible, distributed, large-scale brain networks. While regions within each largescale network are fairly temporally coherent, later studies have shown that each distributed network can be split into a group of spatially granular, and temporally covarying functional parcels. Thus, higher model order ICAs (75~150 components) have been employed to identify functional units known as intrinsic connectivity networks (ICNs). Our recent work suggests that an ICA framework can identify even more granular and functionally homogeneous brain functional units, and has the potential to provide more precise estimates of ICNs. In this study, we adopted an ICA with 1000 components (1k-ICA) to parcellate the brain into fine-grain sparse but overlapping ICNs and evaluated their properties and reliability in various ways. Our findings show that ultra-high-order ICA approaches like 1k-ICA can provide reliable, spatially-sparse ICNs.